Friends, we are nearing the end of the heating season, and I was just browsing through the data (taken every two minutes through the last year!) and I wondered how the temperature in the middle of my house had varied through this last winter. The answer is that the temperature through the winter has stayed at a remarkably stable 21.5 ± 0.4 °C. Allow me to show you the data.
The whole data set

Click on image for a larger version. Graph showing the variation of room temperature in my home over the last year. The graph shows more than a quarter of a million data points. Also plotted is the variation in external temperature shown as a thin line and also the daily average temperature shown as a thick blue line.
Notice that last summer the internal temperature rose as high as 25 °C when the external temperature rose to 30 °C during the day. But in winter, the temperature stayed relatively stable even when the external temperature fell below 0 °C.
Aside from the temperature we can also plot the heat delivered by the heat pump.

Click on image for a larger version. Graph showing hourly averages of the heating power delivered by the heat pump.
In summer the heat pump is used for delivering hot water, but in winter the heat pump also delivers heat for space heating at a rate of roughly 1.5 kW (i.e. 36 kWh/day), with a peak heating of 3.6 kW in January’s cold spell. Let’s place the previous two graphs together.

Click on image for a larger version. The upper part of the graph shows the variation of room temperature and external temperature in degrees Celsius and the lower part of the graph shows heating power in kilowatts.
Putting these two graphs together we can see clearly the correlation between heating power and heating demand – the difference between internal and external temperatures. We can see that on the coldest day the maximum hourly average of the heating power was ~3.6 kW when the heating demand was approximately 23 °C ± 2 °C. This gives us an estimate for the heat transfer coefficient of the house as 3,590 W / 23 °C = 156 ± 12 W/°C.
Winter
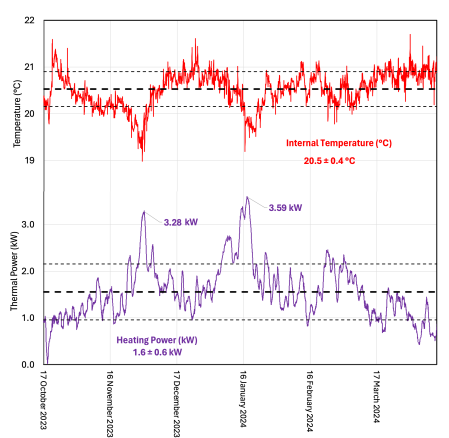
Click on image for a larger version. Data from the winter of 2023/24. The upper part of the graph shows the variation of room temperature and external temperature in degrees Celsius and the lower part of the graph shows heating power in kilowatts. Also shown are the averages of the data over the period shown.
Looking more closely at the winter data four things occur to me.
- The data are not bad. Most of the time the internal temperature was in the range 20.5 ± 0.4 °C – and the house was comfortable all winter.
- However, the actual set temperature was 20 °C. And in the coldest weather the internal temperature fell to between 19 °C and 20 °C. The heat pump has more than 5 kW of available power at 0 °C, so I am not sure why it didn’t compensate to keep the internal temperature stable. This is a failure of the control system and I will be
twiddling some settingscarrying out investigations to try and improve this for next winter. - The average heating power over winter was roughly 1.6 kW which is just 32% of the nominal heating power of the pump. Sadly, like most heat pumps, the Vaillant Arotherm 5 kW can only smoothly alter its output down to 40% of its nominal capacity. In other words, most of the winter it has to switch on and off – typically once an hour – in order to deliver heat at the required rate.
- Finally, the average heating power over winter was roughly 1.6 kW or 38.4 kWh/day. At an average COP of 3.5 this corresponds to about 11 kWh of electricity per day. We filled the battery (capacity is just over 12 kWh) with electricity at 7.5 p/kWh during the night, and then ran using the battery until it ran out. We then had to buy some full price electricity at the end of each day. But during December and January heating costs were still just ~£3.50/day.
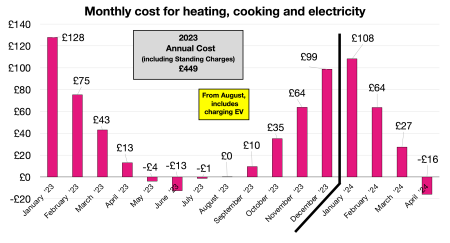
Click on image for a larger version. Monthly costs for electricity over the last year. The cost for April 2024 is an estimate.
April 20, 2024 at 6:22 pm |
Hi Michael
Thought provoking stuff as always! While I mull over what might turn into questions relating to one or two more detailed aspects, one quick question has occurred to me…
If I recall correctly, your heating system controller relies on “weather compensation” (which you’ve described in a previous episode) – i.e. an algorithm which effectively sets the heating power (in fact it controls the the heat pump’s flow temperature) based on the outdoor temperature.
Doesn’t this mean that the correlation you refer to is a direct result of how the controller works?
If so, then what is interesting is your initial observation, regarding how the indoor temperature is (at least during the heating season) more or less constant, and not very different to the target value. I feel sure you must have plotted indoor temperature vs outdoor temperature and would be interested to see how that looks. My guess (based on your first plot, which shows those two quantities vs time) is that it will show a fairly strong correlation with a slope of the order 0.2 … perhaps that can be turned into a recipe for twiddling those settings?
Best wishes
Simon
PS For those of us with non-weather compensation-based controllers, the (more or less) constant indoor temperature is the thing that is (or should be) true by design, because the controller works off the measured indoor temperature, and an observed correlation between heating demand and indoor-outdoor temperature difference is, arguably, the thing of interest. Unless that indoor temperature isn’t as constant as it should be…
April 20, 2024 at 8:10 pm |
Simon, How lovely to hear from you. I trust you are well.
The correlation between heating demand and heating power would be the more-or-less the same independent of the control algorithm. I could use an on-off controller with fixed flow temperature and get better control but the efficiency (i.e. COP) of the heat pump would suffer and that means it would cost more and emit more CO2.
The question for me is why doesn’t the weather compensation work perfectly? In fact I have weather compensation based on outside temperature (which is equivalent to a P-like term in a PID circuit) and also some custom setting in the arcane menu which I think works a bit like the I term in a PID controller.
Anyway. I just thought it was an interesting graph. I’ll change some stuff this summer and see if gets better!
Best wishes for your own endeavours
M
April 20, 2024 at 9:40 pm
I’m well, thanks – you too, I trust.
Perhaps I expressed myself badly – I was thinking not so much of the algorithm as the input that the controller works with.
If the input is the “error”, meaning the discrepancy between the target and a sensed value (indoor temperature) then there is the possibility for feedback, and the controller can show self-correcting behaviour (if e.g. PID parameters are appropriately “tuned”).
But if the input is the outdoor temperature then, without some other information (a matter of “calibration” and/or choosing appropriate parameter values) the controller has no knowledge of what the indoor temperature might be, only what the target value is, and an “error” can’t be defined. Self-correction won’t happen unless parameters have appropriate values by chance.
Perhaps you could clarify why it is that you expect the weather compensation to work perfectly? That would seem to require that the controller has some representation of the relationship between outdoor temperature and overall heat loss. Where does that come from, in the absence of any sensing of indoor temperature?
BW
April 21, 2024 at 10:25 am
Good Point. Different heat pumps operate differently, but there is a heat loss curve which is set by the installer (and adjustable) which specifies Power versus Outside Temperature. Initially the curve is set to match the calculated heat loss (kW) at the design external temperature (~-3 °C). This will need to be adjusted by the homeowner.
The system doesn’t take account of solar gain which can be a very significant effect.
Does that make sense?
M
April 21, 2024 at 12:48 pm
Thanks, Michael – yes it does make sense, and I’ve just reminded myself of such matters by looking at the “heating curve” section of my boiler manual, where it describes its version of weather compensated operation.
(My installer was a bit unenthusiastic about this option, aware that it doesn’t play nicely with the third party control system he recommended. Eventually, I found that limiting the output to 7 kW and leaving that third party controller to do its TPI/on-off thing, produces a remarkably similar overall behaviour, with a flow temperature rising and falling according to the demand for heat but never getting anywhere close to the “set” value, which is 60 degC.)
That manual offers suggestions for adjusting the slope of the curve according to whether the owner has under-floor heating, over-sized or normal-sized radiators but, beyond that, it must be a matter of trial and error to improve on whatever value is chosen at commissioning.
The physicist in me is disappointed that everything is expressed in terms of flow temperature rather than heating power output – I take it that the curvature of the heating curves is ultimately due to the non-linear variation of emitter output with temperature (elevation), and they would become straight lines if only they were converted to plots of power output.
Overall though, I learned that there are essentially two parameters, the slope and the “level”, and I would suppose that adjusting the slope could eliminate the correlation between variations in indoor and outdoor temperature, while adjusting the level should deal with any offset between average actual indoor temperature and the set value. What we would both recognise as a process of “calibration” 🙂
Cheers
April 21, 2024 at 8:17 am |
Hello Michael, thanks for another interesting write up! I do agree with you that the I-like term is much of the problem here. I find myself wondering if there is a hidden and un-adjustable time constant associated with the room temperature modulation, which seems too long for the simple ‘Active’ setting to do very much. Increasing the heat curve value helps, I find, but then causes the room to be too warm when the weather is mild.
For me, the best compromise has been a slightly raised heat curve and then use the ‘Expanded’ setting to cut the heating if the sun comes out. I also tried the adaptive heat curve but I found that the controller just walked the curve value up and up until it was essentially behaving as an on-off controller.
I realise this is less efficient, but for me it’s the best comfort-efficiency trade off I’ve found so far. It’s also maybe not as bad as the SCOP (3.8) would suggest because the heat pump does spend more time not running, so the overall increase in energy use may be smaller than the SCOP would imply. I don’t know.
I did also find that the Vaillant outside thermometer was reading about 2C too high compared to a thermometer a few meters away. This seemed to be a local heating effect from being fixed to the house wall under a ledge. Moving it away to a garage wall has brought the two thermometers into agreement.
I’m very much looking forward to you taking a look at the controls!
April 21, 2024 at 10:30 am |
I’m pretty sure that this teh setting which works best for me too.
The problem is one can only do meaningful experiments in winter and one’s partner doesn’t really enjoy “playing” with something as basic as heating.
I hope to have installed new radiators by next winter which will hopefully lower the flow temperature and improve the COP. And maybe improve temperature control?
Best wishes: M
April 21, 2024 at 8:21 am |
Hi Michael, so good to see another intriguing data-rich post from you, I hope you’re doing well!
I’m with BW, I don’t understand why you’d expect your heating system — no matter how carefully you tune it! — to keep your house’s temperature nearer than a degree (or two!) of its set-point. That’s an excellent level of temperature control IMHO, and if you did better than this, would you even feel it?
“We found a very clear result: temperature change is an immediate perception, and our sensitivity threshold is +/- 1°C. The variability between participants was very small, despite they were different in many psychological dimensions and they described themselves as more sensitive to the cold or to the warm, or as aware of their body or not.” (https://www.climateforesight.eu/interview/yes-you-can-feel-one-degree/)
Anyway, it seems you’re measuring temperature at a single point in your house. Other points will surely be at temperatures that are more than a degree away from 20 degrees C… and if anyone in your household is feeling significant discomfort at any of these points, then maybe your time would be better spent on figuring out how to reduce those temperature variations *within* your house, than on improving the (IMHO already excellent) level of winter-time temperature control at *this* particular point in your house?
April 21, 2024 at 9:51 am |
Hi Clark – and apologies for the lazy truncation of my sign-off in that reply to Michael 😉
That’s an interesting link, but I wonder if our perception of temperature is more complicated than sensing ambient air temperature? I think thermal radiation comes into it too, if only in circumstances where the temperatures of surfaces we can “see” are different from that of the air around us. Quite how we balance the two is unclear, but I’ve felt for myself how effective IR panel heaters can be, so thermal radiation certainly counts for something.
Unlike my former colleague Michael, I live in a house that has both poor insulation and poor airtightness (mid-19th century, solid brick walls, and conservation area constraints which preclude most courses of remedial action). The relevance of this is that in my case, there is much to be gained by monitoring temperature in multiple (even all) rooms and I’m quite used to seeing differences of a few degrees, whereas in Michael’s house with its external wall insulation and better airtightness, I wouldn’t be surprised if multiple sensors reported much more closely similar readings.
But I’d still assume, probably naively, that the sensors report something directly related to how warm I would feel.
Best wishes
Simon
April 21, 2024 at 10:42 am |
Clark, Good Morning. Yes, I am well and inspired by the occurrence of a few sunny days.
I agree with all your points, but it would be boring to end my reply there.
In fact I chose not to include data on the temperature distribution within the house because it is not data-logged but every time I have checked it, it is with 1 °C of the thermometer in the central room. I don’t know specifically why it is so close, except that the house is well-insulated.
On the cold days, my wife and both felt a little cold and wore warmer clothing in the house. Not exactly a disaster. As I mentioned to Simon in this comment chain, the temperature adjustment doesn’t take account of solar gain or windiness, both which affect the proportionality between “required heating power” and “outside temperature”.
So perhaps in the cold months I could shift to on-off control based on a thermostat with manual setting of flow temperature. Mmmmm.
Best wishes
M
April 22, 2024 at 6:44 am |
An interesting discussion! If I were modelling this heating system experimentally (and had housemates willing to be “test subjects”), I’d perhaps start by trying to figure out how best to estimate the time-lag between a change in the heat-pump’s wattage and a change in the inside-outside temperature differential. *Possibly* this would allow me to estimate the thermal mass of the household… but then again I’d not be confident I could devise a valid linear model (for which an easy-peasy multiple linear regression on a timeseries of observations could give valid results; I’d be concerned about collinearity between the conveniently-measured primary factors of wattage and temperature differential. But! I won’t do a performance analysis without a performance goal in mind, and trying to get the inside temperature of any household (not even my own) stable to better than +/-0.5 degrees C wouldn’t motivate me!
And … I believe there’s ample scientific support for Simon Duane’s hypothesis that our human “perception of temperature is more complicated than sensing ambient air temperature”. I believe there are at least a few other factors that are at least as important as an ambient air temperature (as measured by a thermocouple). For just one example: you can also measure a “wet bulb temperature”, see e.g. https://en.wikipedia.org/wiki/Wet-bulb_temperature for a muddled discussion of the concept and its effects on human perceptions of comfort. The article I had linked in my previous comment pointed to a few other factors which *might* turn out to be very important. ”We would also like to study the influence of other senses by adding auditory and olfactory stimuli: some research shows that if you hear the sound of water, or smell the scent of mint, you are prompted to feel cooler. Imagine offices in summer with water falling sound in the background, a scent of mint, and cold lights!” Some folks have noticed that a 20 degree ambient temperature feels quite chilly to them in mid-summer, but quite warm in mid-winter. My personal theory about this was that, after another wonderful beautiful (but cold cold cold!) winter walk at -20 degrees in Duluth Minnesota, a house at 20 degrees feels very very warm…. whereas on the (few!) summer days when the outside temp is above 30, a house at 25 is as cold as I’d want it to be!
All to say that I believe a “comfortable temperature” is inherently a subjective perception, and is not something that can be measured objectively.
April 22, 2024 at 9:51 am |
Clark, Good Morning, and thank you for thoughts which I think are focussed on two areas
Firstly, as regards the delayed action of heating by a heating system and the fall in outside temperature, I think that this may be a possible advantage of a weather compensation system. When the outside temperature falls the heat pump immediately increases its output without waiting for an actionable fall in temperature internally. I could imagine this might give a more stable internal temperature without having to program in a response time constant.
Secondly, regarding the subjectivity of a “comfortable temperature”, since this is an internal human sensation, I agree that it is not something which can be measured objectively. In my previous employment at NPL the temperature department were asked to intervene in an Office War about air temperature. One faction thought it freezing and the other perfectly adequate. Distributing temperature loggers around the office we found that it was remarkably stable and consistent 20 °C everywhere in the office. As you say, determining a “comfort index” involves measurements of radiant heat, humidity and air flow – and perhaps mint-iness too! – and also depends on the level of activity, clothing and amount of subcutaneous fat.
And of course what we consider comfortable now in the UK – 21 °C ish – would be considered quite extremely hot by a previous generation who despite their grievous suffering of 19 °C homes, seem to have survived.
Anyway: as we enter summer I see another heating season on the horizon. What experiment shall I perform this year?
Best wishes. And stay warm. Or cool.
M
April 23, 2024 at 4:21 am
Good point! I see very little “lag” in your dataset, and it might well be that your system’s “weather compensation system” is doing such an excellent job of avoiding overshoot and undershoot that a careful analysis would show no room for improvement on that score. And anyway, since it’s already doing so well at temperature control… perhaps your next “experiment” should attempt to correlate the *perceptions* of discomfort that you feel this next heating system, with any other objective timeseries (such as relative humidity, barometric pressure, daily activity as recorded on a smartwatch?) that you can conveniently collect? You might be able to recruit another test subject within the constraints of experimental ethics, and the no-doubt-tighter constraints of preserving domestic harmony!
And… I’m amused to hear that NPL had a “temperature department” — suggesting to me that the folks working in that department (or at least their managers) had a one-eyed focus on this (easily measurable, and moderately-controllable) metric, rather than a holistic view of office “comfort”. I can well imagine that the NPL’s “Office War” ended in, at best, an uneasy truce between the factions which felt “too hot” and the ones who felt “too cold”. I’m also reminded of the tendency of any modern manager to select a set of KPIs which are measurable, and which they expect to be economically manageable — without much (if any) periodic review of their validity for improving (or even measuring) “what really matters” to any stakeholders who aren’t thoroughly invested in the status-quo of the *existing* set of KPIs and how “their” organisation’s behaviour is responding to these. NZ’s current government is a *big* believer in having a small set of measurable KPIs, see https://www.dpmc.govt.nz/our-programmes/government-targets, sigh. But as usual I digress!
Back to the subject at hand… after a bit more reflection, I realise I’d find it extremely challenging to build a model of the thermal behaviour of your house that has just six independent variables (two successive measurements of inside & outside temp, and one wattage measurement), and a few “hidden” parameters (such as the thermal mass of your house) that’d have any chance of being a good predictor of the next inside temp reading.
I have only a little experience with building a thermal model — and it was unsuccessful! About six months ago, I tested a few models for the thermal behaviour of the inverter on my solar system, using as inputs the time-series measurements of its temperature and output wattage. I then added a few more variables, in an attempt to deal adequately with its *highly* nonlinear behaviour on cloudy days, especially in late afternoon. My goal was to find a reasonably-accurate (albeit indirect) way to measure to my inverter’s current “effective” series resistance (in a two-resistor model of its electrical behaviour), in the belief that this would be a reliable proxy for the passivation of the high-current semiconductor junction(s) which cause any inverter to run hotter and hotter as it ages. Unsuccessful! This inverter is passively air-cooled, so its ambient temperature is important — and I didn’t have a time-series measurement of this. Moreover I wasn’t at all confident of my thermal model. And I finally concluded that it was *much* more interesting, as well as much more do-able, to characterise the “searching inefficiencies” of my inverter’s MPPT at finding even a vaguely-optimal operating point, during the not-uncommon highly-variable cloudy periods of the sky at my location. See https://cthombor.wordpress.com/2023/11/26/mppt-is-problematic-for-my-domestic-rooftop-pv-in-auckland/ if perchance you’re interested!
April 23, 2024 at 10:07 am
Clark, Good Morning.
Three things:
1. Yes NPL had a temperature department and a pressure department etc: it was historically organised around techniques of measurement rather than application areas combining multiple techniques. Many managers have tried to re-arrange things in terms of multi-technique application areas, but it turns out that if you want to be the very very best of something, then you need to obsess about that thing.
2. Regarding KPIs in the years before I left NPL, a management initiative established 6 (I think) kPIs. They put them on every piece of stationery and the web site and every thing we did had to be justified in terms of which KPI us was directed towards. After I left it was reported to me that every single KPI was missed and the scheme was scrapped and the whole incident sank beneath the waves of the sea of failed management initiatives.
3. The “lumped thermal model” which involves a thermal resistance R, a heat capacity C, and a time constant RC is phenomenal useful in all areas of physics as well as electronics. But in physics, when one looks in detail, there are nearly always other terms: parallel heat loss paths with characteristic Rs and Cs. Lumped thermal models are readily soluble but adding further parameters renders them insoluble without prior knowledge of the likely magnitude of the terms involved. So modelling an MPPT algorithm with internal power changing constantly depending on inflation which affected inverter temperature and external temperature sounds… hard.
But building physics is almost entirely built upon static models in which the C term doesn’t matter. And these are really quite linear in reality. Extending to the dynamic thermal models and things get complicated very quickly, because the RC constant for the air and inner surface of the walls is generally much shorter than the RC constant for the house structure.
Anyway: I’m sure you knew that already.
Best wishes: M
April 23, 2024 at 7:16 pm
Call me naive, but I think that a fairly simple model should be able to capture much of the dynamics in domestic heating. There are two very different timescales: (i) how long it takes for indoor air to be exchanged for fresh air – perhaps 2 or 3 hours, based on Michael’s measurements, and (ii) how long it takes for heat stored in the brick walls to transfer in or out – perhaps 2 or 3 days, based on my own experience of turning the heating off when I was away for a week one January.
On Michael’s coldest day, when the heating power peaked at 3.6 kW, I think (combining information from this and an earlier blog) that about 1 kW went into heating the air that leaks out, and so only 2.6 kW would have been conducted through the walls. The immediate response of a weather compensation-based controller is appropriate for heating the air, but my intuition would be that perhaps 2/3 of the variation in heating power should be lagged by (?) 2 or 3 days. I admit that actually demonstrating that by an analysis of observed data might not be easy, because outdoor temperatures are often reasonably stable over a period of 2-3 days. But I’m inclined to try …
Perhaps it’s evident that, just as Michael enjoys few things more than experimental investigations (unconstrained by thoughts of KPIs and the need for Results), I have that reaction to “modelling” – especially when it involves the heat equation!
Best wishes to you both
April 24, 2024 at 4:13 am
Correction: a suitable RC-tree model for Michael’s domestic-heating system might have two leaky capacitors (composed of a capacitor and a shunt resistance) and one series resistor. Simon, would that be the usual way to model a thermal system that has two time-constants?
April 24, 2024 at 9:57 am
To be honest, my thermal modelling always started from approximations to the underlying physics (how does energy flow through the system, and can I identify important routes of transfer between identifiable components, etc) and I would ask myself: what do the slowest modes look like?
I’m fairly sure that this way of thinking is essentially the same as Michael’s “lumped thermal model”, but I don’t start with observed time constants and cook up a model that matches that behaviour – rather, I ask what time constants should I expect to see in a plausible approximation to the system as I understand it.
In cases where it’s not so obvious that there really are only a few more or less separate components, with distinct routes/channels of energy transfer, I’d quite happily resort to the numerical solution of a finite element approximation to the underlying heat equation. Professionally, I had the advantage that in my application (not buildings!) convection was never important, only conduction (with small amounts of radiative transfer to which a linear approximation was close enough).
Being linear, such a system can always be analysed in terms of normal modes which behave independently of one another. If there is a smallish number of relatively slow modes, then taking these on their own can be a way of deriving, or at least motivating, a lumped thermal model.
In the case at hand, I think the identifiable components are the enclosed indoor air and the brick walls (including a boundary layer between the bulk of the indoor air and the inside surface of the brick walls and a layer of EWI separating the outside surface of the brick from the outdoor air). The energy transfers are from the heating system into the indoor air, from the indoor air into the wall, from the wall to outdoors, and the ventilation/infiltration loss by the exchange of indoor/outdoor air. The significant thermal masses are the wall and the indoor air (I’m sure the boundary layer and EWI have negligible thermal mass). The heating system injects energy into the indoor air at some rate P(t) and the outdoor temperature T_out(t) varies somehow. The first question I would ask is how the (assumed spatially uniform) indoor air temperature T_in(t) responds when P(t) and T_out(t) take various forms – that should reveal the dynamics, and also suggest ways in which P(t) can be made to respond to T_out(t) with the goal of achieving some target value for T_in. That is, a heating control system algorithm.
At some point, curiosity about such a model will overcome my laziness and I’ll find out how well it does for my own leaky house, with its rather poor insulation…
Cheers
April 25, 2024 at 3:15 am
Thanks Simon, that’s interesting — and I’m sure it’s a valid approach to the problems you try to solve with your modelling! Most of my experience is with modelling computer systems which are far far too complex to be modelled effectively as a composition of components whose measurable behaviour is well-described by physical laws. So: Occam’s Razor is always in my hand, I shift to a more complex model only after I have discarded all of the simpler ones I could think of, and after I get an acceptably-good fit using a complex model I *always* look for a simpler model which is also acceptably-good. *Sometimes* my models are “only” descriptive (as in models of personal identity, privacy, or security ); sometimes they are purely analytic (in cases where there’s a well-accepted model that hasn’t yet been reduced to an analytic one); sometimes they are statistical (e.g. I sometimes perform a linear regression on a set of measurements whose deviations from linearity are “obvious” but still quite a bit smaller than the “noise”… as in a recent study of the evolution of the state-of-health of the batteries of various models and model-years of the Nissan Leaf). All to say that I’m heavily biased toward simplicity in my models, so when a simple RC model doesn’t suffice (or rather, when observations of the “output” don’t fit nicely with an exponential-decay response to a change in “input”), and someone mentions a second time-constant may be a good first-approximation to the *error* in the simpler model, I think about a model with four or five parameters in a functional form which — conceivably — could be “fit” statistically to the observations.
Horses for courses, as always!
April 25, 2024 at 8:06 am
Indeed “modelling” has a range of meanings and I recognise the benefit of adapting one’s way of thinking to context. If the underlying aim is to enable predictions based on trends in past and current behaviour, then that “as simple as possible, but no simpler” approach seems optimal. Once the behaviour has been captured in such a (phenomenological) model, the question may then arise of whether that behaviour could somehow be “improved” – that’s when it helps if the model and its parameters have some interpretation. But, as a theorist by training, I usually struggle to resist such a way of thinking …
I wonder if our exchange is drifting uncomfortably far from Michael’s original topic “My Room Temperature” to justify taking up space in the comments – I’m sure Michael will let us know if it would be better continued elsewhere 😉
Cheers!
April 26, 2024 at 2:42 am
Yeah, this comment stream is an awkward venue for a nerdy conversation about modelling! I too am a theorist at heart, but I’m a “practical theorist” — I have no interest in working on “elegant” models that have no empirical grounding, nor do I have any interest in models which vaguely approximate empirically-measured behviour but which lack any theoretical explanation. However I get excited when I see a nicely-fitting behavioural model that lacks a theoretical “explanation”, or a theoretical model that hasn’t yet been “reduced to practice” (or, more often, not surviving its “first contact” with reality) by fitting it to empirical data.
Case in point: I fondly recall hacking assembly code (on a model-33 Teletype, with papertape IO!) for one of the first commercially-successful FT-NMR instruments, while an undergraduate… the guy who hired me (and who wrote the assembly codebase I extended hugely) had a short-and-sweet bit of code that’d assign a “peak value” to a series of points which were (at least to the human eye) recognisable as a Lorentzian signal with a somewhat-Gaussian shape due to filtering. I puzzled over that for a while, and finally decided that the peak-height (as estimated from three samples) would be accurate for a triangle-shaped waveform. I eventually wrote (in assembly code, yikes!) a hack that’d reliably converge on a least-squares fit to a Lorentzian; and then it was easy-peasy to calculate the height *and* area of that Lorentzian. My code could also be configured to do a LL fit to a Gaussian — and that was more appropriate for lightly-filtered NMR plots. I couldn’t figure out how to reliably converge on what I “knew” (from theoretical considerations) to be a Gaussian-filtered Lorentzian signal… anyway I’m pretty sure I set the default to Gaussian. In the real world of signal processing, it’s rarely the case (in my experience) that we do much more than apply some heuristics of dubious (or non-existent) theoretical grounding! Contemporary FT-NMR has, I have the vague sense from my google search just now, pretty much settled on a heuristic but easily-computed measure called the “peak width at half-height” and an *assumption* that all peaks are Lorentzian. https://www2.chemistry.msu.edu/courses/cem845/FS21/DH%20NMR%20Basics_17.pdf
So many real-world problems a modeller *might* address! So little demand for a more-accurate more-theoretically-grounded modelling! And… our world’s increasingly time-pressured, resource-limited, not-terribly-analytic decision makers increasingly rely on hastily-hacked not-fully-quality-assured tables and graphs. Sigh. I must sound like a grumpy old man 😉
April 26, 2024 at 10:27 am
Clark, you don’t sound grumpy. And it’s interesting to see the problem of fitting a mixed gaussian and lorentzian peak emerge in another place: it’s fundamental to spectroscopy and a colleagues spent quite some time working on technique for very rapid fitting of such peaks.
I should say that Simon’s interest in fitting transients stems (as far as I know) where he built a super sensitive micro calorimeter for estimating absorbed radiation dose. Since it was a primary measure – he had to start with a model he really believed to be close to physical reality – because it was the parameter estimation he wanted to extract. He wasn’t really at liberty to ‘tweak the model’. (Simon: have I understood that correctly?).
In any case, it’s interesting to see how much our professional lives have been spent on such similar tasks in such different fields!
Best wishes: M
April 26, 2024 at 3:40 pm
Yes indeed, Michael, that’s the essence of it. Your comment is also helpful because it explains why, in fact, my interest was always in absorbed energy, and whether I could account for it all. This was rather taken for granted in my comment about how I approach modelling. In measuring dose, although we would easily talk as if we were sensitive to temperature changes of the order a few micro K (hence the “micro” in the name that device was given), in practice temperature was for us only an indication of where the energy might be going. I don’t think we really needed to know absolute temperature to better than several mK. I’m also reminded of a fleeting moment when it seemed there was a possibility that in our attempts to improve the dose measurements we might get help from you directly. I remember we had an initial discussion, but I can only guess that people with influence decided that it wasn’t to be, which was a great shame as far as I was concerned.
One of the reasons I’m enjoying the wide-ranging exchange here is the unexpected turns it takes – prompted by Clark’s comment, I’ve just educated myself about the Lorentzian/Cauchy distribution (I never needed to know before, and couldn’t muster any interest in all the talk of Breit-Wigner resonances in scattering phenomenology when I was a graduate student). Which is a slight shame, because that distribution has the kind of mathematical properties that would undermine people’s intuitive grasp of statistics if only they knew – and that’s just the kind of thing that *does* interest me.
Cheers both
April 22, 2024 at 7:00 am |
Here’s a less-muddled and much-more-informative Wikipedia article on the importance of factors other than dry-bulb temperature in the human *perception* of comfort. https://en.wikipedia.org/wiki/Thermal_comfort
April 23, 2024 at 12:23 pm |
Thanks for that – I’d say it’s almost overwhelmingly informative, in fact – but it did lead me to clarification on https://en.wikipedia.org/wiki/Mean_radiant_temperature, and introduced me to https://en.wikipedia.org/wiki/Operative_temperature, which looks like a good answer to my question of how to balance various different influences when it comes to our thermal comfort.